AI Trends in 2025 and Human Centered AI
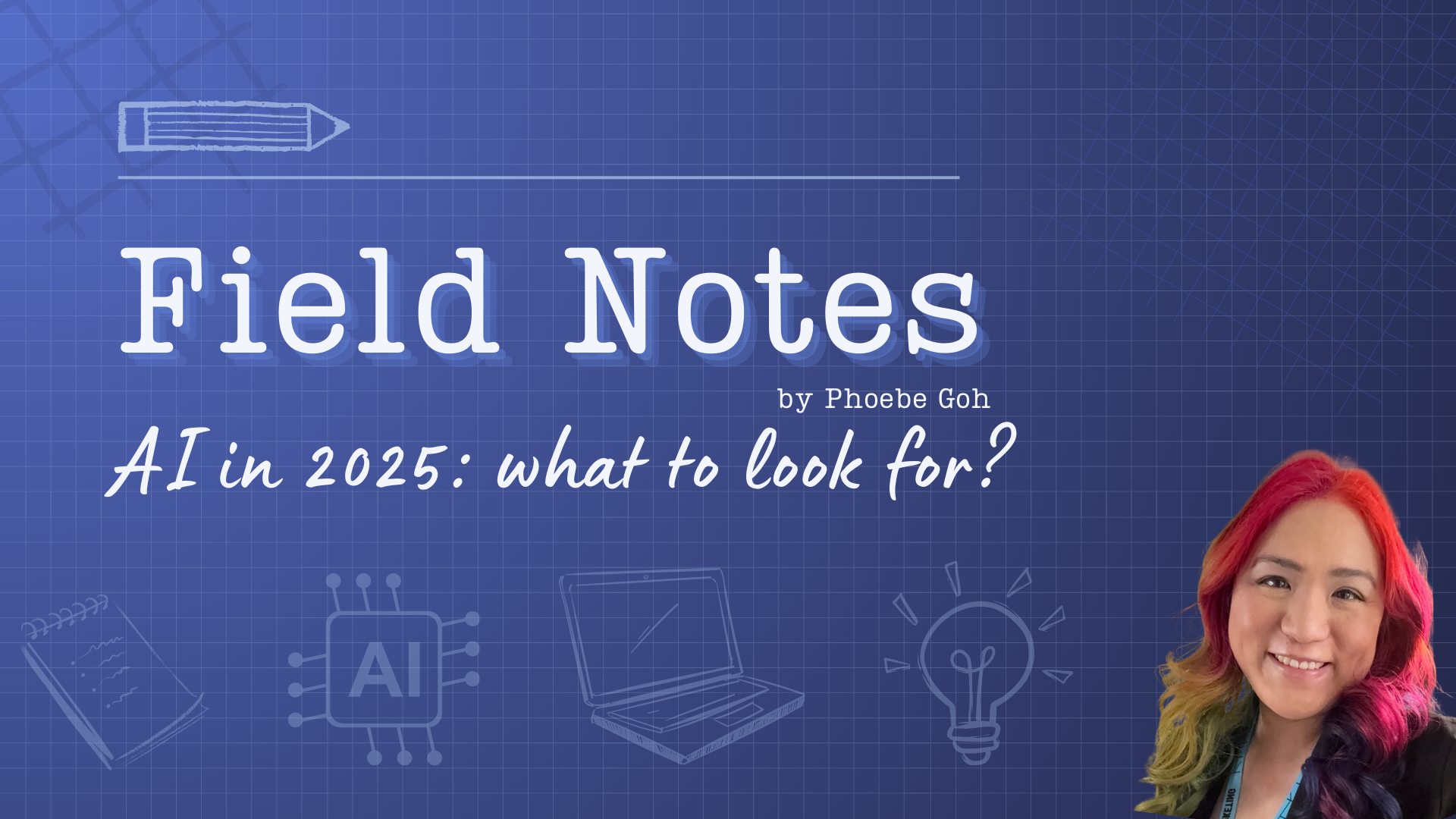
Field Notes: January 6, 2026
Like everyone in 2025, we’re going to spend a lot of time talking about what’s going on in AI right now. For a field that is rapidly changing, that means we’re seeing the evolution of the technologies themselves as well as how AI should be implemented.
This week I’ve picked two articles that caught my eye - one looks at the technology, one looks at the usage. A ‘balanced’ way to start the year! Check out my opinions at the end.
Source 1: Trends in AI
Firstly, 7 AI Trends and Predictions for 2025 Everyone Should Know! (Pavan Belagatti) is a summary of what’s coming in the new year in terms of technologies. While many of these concepts have been around for a while, we’re only just starting to see organisations scratching the surface of what they’re capable of.
Belagatti covers seven big trends in AI at a high level, including:
- Agentic AI: coordination and execution of multiple tasks and decision-making
- Multi-agent RAG: adding multiple agents to RAG systems to improve performance and provide more accurate responses
- AI Framework maturity: more sophisticated frameworks to decrease time to market for new AI applications
- Securing AI systems: (this should be higher on the list, I reckon!)
- Small Language Models: Lower operational costs, faster inference times. What’s not to like? Will be more popular in 2025 as AI continues to become more mainstream.
- LLM Cost Optimization: Balancing performance and cost - new strategies to prevent resource waste, distribute processing, and continuous optimization of AI systems.
- Databases’ role in GenAI: More databases will be “AI-ready” and they’ll all claim to be the best for GenAI.
Source 2: Human-centered Artificial Intelligence
The other article that came across my inbox was this one from McKinsey, The case for human-centered AI (McKinsey).
It’s a transcript from the McKinsey podcast, At The Edge, between McKinsey senior partner Lareina Lee and Professor of Computer Science at Stanford and cofounder and codirector of the Stanford Institute for Human-Centered Artificial Intelligence (HAI), James Landay.
Lee and Landay discuss why taking a human-centric rather than technology-centric approach to generative AI will be required to maximize its potential.
Some takeaways:
- Landay once said “‘AI for good’ isn’t good enough” - if it’s not done in a human-centric way, you won’t be successful in achieving that ‘good’.
- Human-centric AI includes how AI is designed, created, and used. It requires an interdisciplinary approach that leads to new ideas and new ways of looking at things.
- AI systems are based on probabilistic models - they’re harder to design, and harder to protect against error, than deterministic models, where the correct output can be reliably determined.
- Responsible AI attempts to address questions about how to create models that don’t do harm - but it’s difficult when models are controlled by a few large organizations and others can’t see how they were built or trained.
- Guardrails and blacklists are the most common way we’ll see companies protecting against wrong answers (like hallucinations) especially when using their own data. We’ll look at what the differences are in another article.
- A mix of improved design process, education, and law and policy will be needed to effectively ensure that AI is used in the right ways.
- Landay suggests that diversity through three different disciplines - social scientists, humanists, and ethicists - will help catch and solve problems in AI earlier.
- And finally, an optimistic take on the effect of AI on academia: “I think AI is going to change the educational system because it can’t continue to exist the way it does today, which is largely based on rote learning and certain ways of evaluation, which is hard to do with the AI tools out there.”
Phoebe’s perspective
Whether we are optimistic or cynical, AI is firmly established as part of our modern society. While the actual usefulness of various AI applications is still hotly debated, I see the primary question is:
- is AI a general purpose technology, improving overall human productivity on existing tasks?
- or is AI better used in industry-specific tasks that are uniquely innovative and achieve things that humans couldn’t easily reproduce?
The boring-but-probably-right answer is some hybrid of the two extremes. It’ll be exciting to see if 2025 is the year of a specific AI use case that shakes things up significantly! With technologies like multi-agentic AI, RAG and smaller models there are a lot of combinations to be explored by businesses, universities and individuals.
Underlying all of the great innovation in technology, however, is ensuring that the right data is used in AI solutions, and that the checks and balances - from people, to processes, to regulation - are in place to ensure that the “human” is put at the centre of the design process.
What can we do? Three things I’ll be spending time on this year:
- Understanding the foundational models (llama, gemini, ChatGPT etc) and their “best” applications. I’ll also be learning about small models since these sometimes outperform their larger, beefier counterparts.
- Speaking with people using AI as to how they’re implementing responsible AI - and also, whose responsibility it is within the organisation!
- We know that data is the most important part of AI to deliver successful outcomes! I’ll be spending time on breaking down what makes “good” data and “bad” data, as well as the technology and processes we can implement to ensure that we’re getting more of the “good” data for AI!
I’ll be writing a lot about what I learn so that I can help others cut through the noise and hype of technologies like AI-specific databases, multimodal AI, agentic AI and even the different global regulations and policy that will impact businesses and technologists throughout the year!
Your turn!
What is your focus for AI this year? Will you be learning data science, AI infrastructure, or more of the people and process angles? How do you think AI will change your job?